The Role of Artificial Intelligence in Genomics
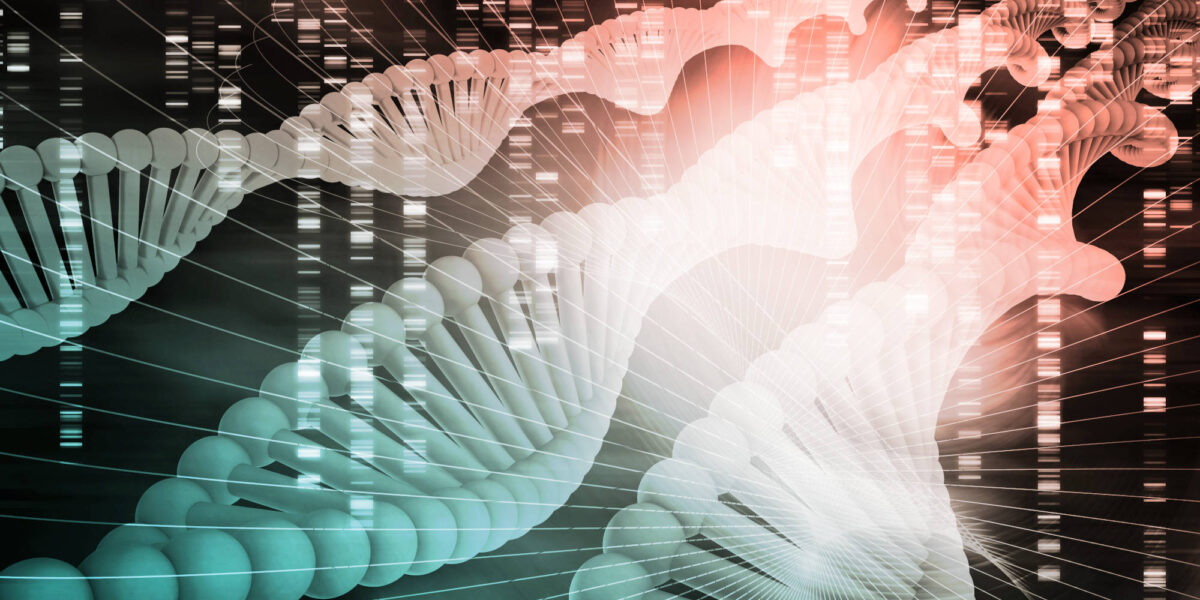
Artificial Intelligence (AI) is defined as the simulation of human intelligence by machines. AI has demonstrated significant utility in genomics, especially within clinical genomics. The application of AI is most relevant as a supplement rather than a replacement for doctors and is often used to rapidly analyze large sets of genomic data1.
The strength of AI interpretation tasks is rooted in the multiple problem classes that AI spans, namely computer vision, time series analysis, and natural language processing (NLP). These three problem classes are often applied to genomics. For example, deep learning (a subset of machine learning) of lung cancer histopathological images is able to identify cancer cells, determine their type, and predict what somatic mutations are present in the tumor. Time series analysis, the analysis of temporal data to forecast future observations, can be applied to genomic sequence data to detect functional DNA sequence elements that are indicative of gene function. NLP involves the computational extraction of meaning from natural human language. When combined with genomic data, NLP-based methods have been used to predict rare disease diagnoses and to drive phenotype-informed genetic analysis2.
In clinical genomics, AI is used to target difficult problems that are not easily solved by clinicians. For example, classification of introns, genes that do not code for protein, is very difficult, primarily because of the complexity of DNA interactions that influence gene splicing, a post-transcriptional modification process in which a single gene can code for multiple proteins. However, AI algorithms are able to give important insights into identifying cryptic splicing variants underlying genetic disorders2.
AI algorithms can also extract higher-level diagnostic concepts embedded in medical images and Electronic Health Records. When linked with genomic data, an AI-based diagnostic agent coupled with a genome interpretation system can rapidly produce genetic diagnoses. This technique, known as AI-based phenotype-to-genotype mapping, could significantly improve the diagnostic yield of genetic testing and the identification of individuals with unrecognized genetic disorders1,3.
There are limitations that need to be considered when using AI, such as hidden biases in the datasets that are used to train AI2. However, AI technology has already been demonstrated to be extremely transformative and useful within clinical genomics.
For example, SOPHiA Genetics developed a genetics AI that uses knowledge-sharing between hospitals to train its algorithms2. 503 hospitals around the world are already using this technology, which can locate and highlight mutations in cancer-linked genes within two hours of uploading raw genomic data. Additionally, the AI platform IBM Watson was able to analyze the genome of a brain cancer patient and suggest an appropriate treatment plan within 10 minutes, while the human experts took 160 hours. These examples represent a microcosm of the potential roles AI machines can play in acute cancer management, relapse management, and management of future cancer risk and risk for relatives.
Sources:
- Greatbatch O, Garrett A, Snape K (2019). The impact of artificial intelligence on the current and future practice of clinical cancer genomics. Genetics Research 101, e9, 1–5. https://doi.org/10.1017/ S0016672319000089
- Dias, R., Torkamani, A. Artificial intelligence in clinical and genomic diagnostics. Genome Med 11, 70 (2019). https://doi.org/10.1186/s13073-019-0689-8
- Álvarez-Machancoses Ó, DeAndrés Galiana EJ, Cernea A, Fernández de la Viña J, Fernández-Martínez JL. On the Role of Artificial Intelligence in Genomics to Enhance Precision Medicine. Pharmgenomics Pers Med. 2020;13:105-119, https://doi.org/10.2147/PGPM.S205082